Balancing data maturity and AI adoption: The struggle between perfection and progress
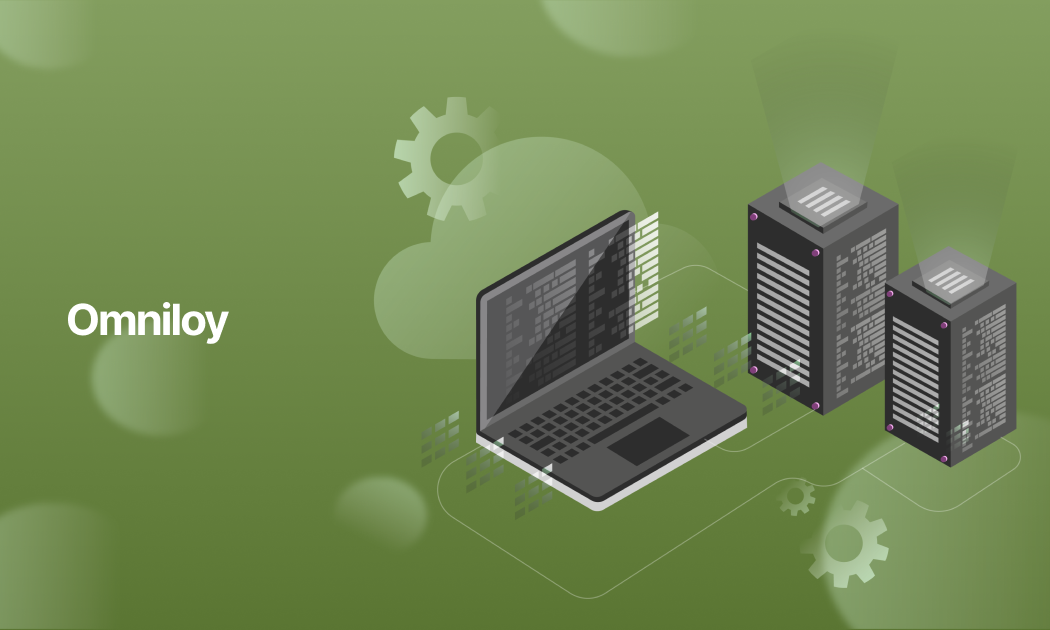
Data maturity typically progresses through these stages:
Data exploration: Ad-hoc data use with limited analysis.
Data informed: Systematic analysis supports decisions, often mixed with intuition.
Data driven: Data guides decisions, focusing on measurable outcomes.
Data transformed: Fully automated data integration for real-time decision-making.
Only 1-2% of companies reach "Data transformed," reflecting the difficulty of this level. Data maturity encompasses aspects like processes, governance, and quality, and achieving full self-service insights remains a major challenge for data teams.
What defines mature data?
It involves key components such as a centralized data warehouse or data lake, along with a robust ETL process for clean and updated data. There are also measures for data quality, governance, and compliance, including regular profiling and cleansing. Cloud-based infrastructure is used for scalability, and secure data-sharing practices are in place. Additionally, an established data team with AI/ML skills, alignment on data-driven insights, and an ethical approach to data management and risk mitigation are essential features of mature data.
Avoid striving for perfection when good is enough
Your data warehouse can still grow while AI enhances data without full uniformity.
Incremental AI adoption: Start small, scaling as maturity grows. Introduce AI in specific areas with strong data quality.
AI to enhance data maturity: Use AI tools for data cleaning and management.
User-friendly tools: Leverage natural language AI to replace traditional BI tools.
Feedback loops: Use AI to improve data quality.
Risk management: Plan for data limitations, ensuring proper protections.
Accept imperfection: Aim for "good enough" data while continuously improving.
If you want to know even more about our product, do not hesitate to book a demo.